An academic is expected to be a jack of many trades – handling research, teaching, mentorship, administration, committee work, reviewing, grant-writing, and editorial duties. Science communication is increasingly being added to that list as well. Outreach, public engagement and science communication are all terms thrown around (e.g. the 'Broader Impacts' section of many NSF grants, for example, includes the possibility "Broaden dissemination to enhance scientific and technological understanding"). Sometimes this can include communication between academics (conferences, seminars, blogs like this one) but often it is meant to include communication with the general public. Statistics about low science literacy at least partially motivate this. For example, “Between 29% and 57% of Americans responded correctly to various questions measuring the concepts of scientific experiment and controlling variables. Only 12% of Americans responded correctly to all the questions on this topic, and nearly 20% did not respond correctly to any of them”. (http://www.nsf.gov/statistics/seind14/index.cfm/chapter-7/c7s2.htm).
Clearly improving scientific communication is a worthy goal. But at times it feels like it is a token addition to an application, one that is outsourced to scientists without providing the necessary resources or training. . This is a problem because if we truly value scientific communication, the focus should be on doing it in a thoughtful manner, rather than as an afterthought. I say this because firstly because communicating complex ideas, some of which may require specialized terms and background knowledge, is difficult. The IPCC summaries, meant to be accessible to lay readers were recently reported to be incredibly inaccessible to the average reader (and getting worse over time!). Their Flesch reading ease scores were lower than those of Einstein’s seminal papers, and certainly far lower than most popular science magazines. Expert academics, already stretched between many skills, may not always be the best communicators of their own work.
Secondly, even when done well, it should be recognized that the audience for much science communication is a minority of all media consumers – the ‘science attentive’ or ‘science enthusiast’ portion of the public. Popular approaches to communication are often preaching to the choir. And even within this group, there are topics that naturally draw more interest or are innately more accessible. Your stochastic models will inherently be more difficult to excite your grandmother about than your research on the extinction of a charismatic furry animal. Not every topic is going to be of interest to a general audience, or even a science-inclined audience, and that should be okay.
So what should our science communication goals be, as scientists and as a society? There is entire literature on this topic (the science of science communication, so to speak), and it provides insight into what works and what is needed. However, “....despite notable new directions, many communication efforts continue to be based on ad-hoc, intuition-driven approaches, paying little attention to several decades of interdisciplinary research on what makes for effective public engagement.”
One approach supported by this literature process follows 4 steps:
1) Identify the science most relevant to the decisions that people face;
2) Determine what people already know;
3) Design communications to fill the critical gaps (between what people know and need to know);
4) Evaluate the adequacy of those communications.
This approach inherently includes human values (what do people want or need to know), rather than a science-centric approach. In addition, to increase the science-enthusiast fraction of the public, focusing on education and communication for youth should be emphasized.
The good news is that science is respected, even when not always understood or communicated well. When asked to evaluate various professions, nearly 70% of Americans said that scientists “contribute a lot” to society (compared to 21% for business executives), and scientists typically are excited about interacting with the public. But it seems like a poor use of time and money to simply expect academics to become experts on science communication, without offering training and interdisciplinary relationships. So, for example, in the broader impacts section of a GRFP, maybe NSF should value taking part in a program (led by science communication experts) on how to communicate with the public; maybe more than giving a one-time talk to 30 high school students. Some institutions provide more resources to this end than others, but the collaborative and interdisciplinary nature of science communication should receive far more emphasis. And the science of science communication should be a focus – data-driven approaches are undeniably more valuable.
None of this is to say that you shouldn't keep perfecting your answer for when the person besides you on an airplane asks you what you do though :-)
Showing posts with label Academic life. Show all posts
Showing posts with label Academic life. Show all posts
Wednesday, October 21, 2015
Friday, September 18, 2015
Post at Oikos + why do papers take so long?
This is mostly a shameless cross-post to a blog post I wrote for the Oikos blog. It's about an upcoming paper in Oikos that asks whether beta-diversity null deviation measures, which originated in papers like Chase 2010 and Chase et al. 2011, can be interpreted and applied as a measure of community assembly. These measures were originally used as null models for beta-diversity (i.e. to control for the effects of alpha diversity, etc), but increasingly in the literature they are used to indicate niche vs. neutral assembly processes. For anyone interested, the post is at the Oikos blog: http://www.oikosjournal.org/blog/v-diversity-metacommunities.
What I found most amusing, or sad, depending on your perspective was that I wrote a blog post about some of the original conversations I had with co-authors about this subject. I looked it up the other day and was shocked that the post was from 2013 (http://evol-eco.blogspot.com/2013/11/community-structure-what-are-we-missing.html). It's amazing how long the process of idea to final form actually takes. (No one phase took that long either - just idea + writing + coauthor edits + rewriting + submit + revise + coauthors + revise = long time...)
What I found most amusing, or sad, depending on your perspective was that I wrote a blog post about some of the original conversations I had with co-authors about this subject. I looked it up the other day and was shocked that the post was from 2013 (http://evol-eco.blogspot.com/2013/11/community-structure-what-are-we-missing.html). It's amazing how long the process of idea to final form actually takes. (No one phase took that long either - just idea + writing + coauthor edits + rewriting + submit + revise + coauthors + revise = long time...)
Wednesday, August 26, 2015
Science is a maze
If you want to truly understand how scientific progress works, I suggest fitting mathematical models to dynamical data (i.e. population or community time series) for a few days.
You were probably told sometime early on about the map for science: the scientific method. It was probably displayed for your high school class as a tidy flowchart showing how a hypothetico-deductive approach allows scientists to solve problems. Scientists make observations about the natural world, gather data, and come up with a possible explanation or hypothesis. They then deduce the predictions that follow, and design experiments to test those predictions. If you falsify the predictions you then circle back and refine, alter, or eventually reject the hypothesis. Scientific progress arises from this process. Sure, you might adjust your hypothesis a few times, but progress is direct and straightforward. Scientists aren’t shown getting lost.
Then, once you actively do research, you realize that formulation-reformulation process dominates. But because for most applications the formulation-reformulation process is slow – that is, each component takes time (e.g. weeks or months to redo experiments and analyses and work through reviews) – you only go through that loop a few times. So you usually still feel like you are making progress and moving forward.
But if you want to remind yourself just how twisting and meandering science actually is, spend some time fitting dynamic models. Thanks to Ben Bolker’s indispensible book, this also comes with a map, which shows how closely the process of model fitting mirrors the scientific method. The modeller has some question they wish to address, and experimental or observational data they hope to use to answer it. By fitting or selecting the best model for they data, they can obtain estimates for different parameters and so hopefully test predictions from they hypothesis. Or so one naively imagines.
The reality, however, is much more byzantine. Captured well in Vellend (2010):
Bolker hints at this (but without the angst):
![]() |
map for science? |
You were probably told sometime early on about the map for science: the scientific method. It was probably displayed for your high school class as a tidy flowchart showing how a hypothetico-deductive approach allows scientists to solve problems. Scientists make observations about the natural world, gather data, and come up with a possible explanation or hypothesis. They then deduce the predictions that follow, and design experiments to test those predictions. If you falsify the predictions you then circle back and refine, alter, or eventually reject the hypothesis. Scientific progress arises from this process. Sure, you might adjust your hypothesis a few times, but progress is direct and straightforward. Scientists aren’t shown getting lost.
Then, once you actively do research, you realize that formulation-reformulation process dominates. But because for most applications the formulation-reformulation process is slow – that is, each component takes time (e.g. weeks or months to redo experiments and analyses and work through reviews) – you only go through that loop a few times. So you usually still feel like you are making progress and moving forward.
But if you want to remind yourself just how twisting and meandering science actually is, spend some time fitting dynamic models. Thanks to Ben Bolker’s indispensible book, this also comes with a map, which shows how closely the process of model fitting mirrors the scientific method. The modeller has some question they wish to address, and experimental or observational data they hope to use to answer it. By fitting or selecting the best model for they data, they can obtain estimates for different parameters and so hopefully test predictions from they hypothesis. Or so one naively imagines.
![]() |
From Bolker's Ecological Models and Data in R, a map for model selection. |
“Consider the number of different models that can be constructed from the simple Lotka-Volterra formulation of interactions between two species, layering on realistic complexities one by one. First, there are at least three qualitatively distinct kinds of interaction (competition, predation, mutualism). For each of these we can have either an implicit accounting of basal resources (as in the Lotka-Volterra model) or we can add an explicit accounting in one particular way. That gives six different models so far. We can then add spatial heterogeneity or not (x2), temporal heterogeneity or not (x2), stochasticity or not (x2), immigration or not (x2), at least three kinds of functional relationship between species (e.g., predator functional responses, x3), age/size structure or not (x2), a third species or not (x2), and three ways the new species interacts with one of the existing species (x3 for the models with a third species). Having barely scratched the surface of potentially important factors, we have 2304 different models. Many of them would likely yield the same predictions, but after consolidation I suspect there still might be hundreds that differ in ecologically important ways.”Model fitting/selection, can actually be (speaking for myself, at least) repetitive and frustrating and filled with wrong turns and dead ends. And because you can make so many loops between formulation and reformulation, and the time penalty is relatively low, you experience just how many possible paths forward there to be explored. It’s easy to get lost and forget which models you’ve already looked at, and keeping detailed notes/logs/version control is fundamental. And since time and money aren’t (as) limiting, it is hard to know/decide when to stop - no model is perfect. When it’s possible to so fully explore the path from question to data, you get to suffer through realizing just how complicated and uncertain that path actually is.
![]() |
What model fitting feels like? |
Bolker hints at this (but without the angst):
“modeling is an iterative process. You may have answered your questions with a single pass through steps 1–5, but it is far more likely that estimating parameters and confidence limits will force you to redefine your models (changing their form or complexity or the ecological covariates they take into account) or even to redefine your original ecological questions.”
I bet there are other processes that have similar aspects of endless, frustrating ability to consider every possible connection between question and data (building a phylogenetic tree, designing a simulation?). And I think that is what science is like on a large temporal and spatial scale too. For any question or hypothesis, there are multiple labs contributing bits and pieces and manipulating slightly different combinations of variables, and pushing and pulling the direction of science back and forth, trying to find a path forward.
(As you may have guessed, I spent far too much time this summer fitting models…)
(As you may have guessed, I spent far too much time this summer fitting models…)
Thursday, August 6, 2015
#ESA100 - EEB & Flow blogging 100 years of ESA
If you are attending, a few links from past years for those last minute preparations:
Presentations
Survival notes
Apparently this was my take-home in 2012
![]() |
I think "postdoc trying to finish their talk" should be added to this... |
Monday, August 3, 2015
Fear of missing out
There are so many tools, techniques, communication platforms and methods out there for ecologists that it can be hard to keep track of them all. Whether it be LaTeX, Linux, or Twitter, someone has probably suggested that your research life will improve if you use their favourite tool.
Online science has allowed fantastic networking and information exchange. However, one side effect of the increased usage of social media can be the feeling that you can’t keep up. The phrase ‘Fear of Missing Out’ in social psychology was coined to describe the anxiety arising from looking at social media and fearing that you have chosen how to spend our time poorly. But this can extend to academic working life too – that nagging fear that your colleagues are doing cooler things (going to more conferences, using more cutting edge techniques, publishing more papers) than you. It can be disconcerting to see that there’s a cool R package you know nothing about, or to receive yet another invite to a work-based social media platform you’ve never heard of.
There are people who are the early adopters and the technological trendsetters; people who derive their success from their methodological/technological skill sets. These people are fantastic, since they provide the examples for others in the field to follow. But for most researchers, it is practical to recognize that knowing every new technique and tool doesn’t promise success.
Graduate students may suffer this fear of falling behind most intensely, given how closely it relates to imposter syndrome and the general skills gap that grad students have to overcome. But I’d argue for most students, that balance is key. Otherwise it can be a slippery slope: maybe you learn R, then find that people recommend knitR and R Markdown, then you learn Shiny to show your results beautifully, then someone suggests things would be faster in a lower level language – and maybe months have gone by. That’s not necessarily bad, intellectual freedom and self-teaching are some of the best parts of graduate school, and if you are going to learn a bunch of new techniques, that is the perfect time. But aficionados will make arguments for everything from Python, Linux, Emacs (apparently the one true operating system), Github, to LaTeX/BibTeX, etc, etc. And every one of these is a useful tool, but maybe not always a necessary tool.
Researchers can never learn everything, and some things will fall out of fashion as quickly as they arose. Sure, it is less than ideal to be a researcher who hasn’t learned a new approach since the advent of SAS. But we need to balance necessity (is it fundamental to my research?), the time investment, and the utility of these skills for future endeavours (e.g. if you are applying for a data analyst job after grad school, learning a few languages might be smart - but if you will rarely use it again, perhaps it is not so important). The danger for many students is that either the time investment in learning a less-than-fundamental tool is very high, or else they learn a number of tools but never master any of them. This isn't to say you can shirk on quantitative skills - on the contrary, having those skills is far more important than mastering any specific program or language. I can think of fantastic ecologists who don’t know what Twitter is, who still use SAS, who have never learned R, and who produce brilliant work. The questions, the ideas, and the knowledge matter too, after all.
(*I should note that I'm a quantitative/theoretical ecologist, and I think every ecologist should have a strong quantitative education! Just that you can do that using lots of different methods and doing it well should always be the first priority.)
Online science has allowed fantastic networking and information exchange. However, one side effect of the increased usage of social media can be the feeling that you can’t keep up. The phrase ‘Fear of Missing Out’ in social psychology was coined to describe the anxiety arising from looking at social media and fearing that you have chosen how to spend our time poorly. But this can extend to academic working life too – that nagging fear that your colleagues are doing cooler things (going to more conferences, using more cutting edge techniques, publishing more papers) than you. It can be disconcerting to see that there’s a cool R package you know nothing about, or to receive yet another invite to a work-based social media platform you’ve never heard of.
There are people who are the early adopters and the technological trendsetters; people who derive their success from their methodological/technological skill sets. These people are fantastic, since they provide the examples for others in the field to follow. But for most researchers, it is practical to recognize that knowing every new technique and tool doesn’t promise success.
Graduate students may suffer this fear of falling behind most intensely, given how closely it relates to imposter syndrome and the general skills gap that grad students have to overcome. But I’d argue for most students, that balance is key. Otherwise it can be a slippery slope: maybe you learn R, then find that people recommend knitR and R Markdown, then you learn Shiny to show your results beautifully, then someone suggests things would be faster in a lower level language – and maybe months have gone by. That’s not necessarily bad, intellectual freedom and self-teaching are some of the best parts of graduate school, and if you are going to learn a bunch of new techniques, that is the perfect time. But aficionados will make arguments for everything from Python, Linux, Emacs (apparently the one true operating system), Github, to LaTeX/BibTeX, etc, etc. And every one of these is a useful tool, but maybe not always a necessary tool.
Researchers can never learn everything, and some things will fall out of fashion as quickly as they arose. Sure, it is less than ideal to be a researcher who hasn’t learned a new approach since the advent of SAS. But we need to balance necessity (is it fundamental to my research?), the time investment, and the utility of these skills for future endeavours (e.g. if you are applying for a data analyst job after grad school, learning a few languages might be smart - but if you will rarely use it again, perhaps it is not so important). The danger for many students is that either the time investment in learning a less-than-fundamental tool is very high, or else they learn a number of tools but never master any of them. This isn't to say you can shirk on quantitative skills - on the contrary, having those skills is far more important than mastering any specific program or language. I can think of fantastic ecologists who don’t know what Twitter is, who still use SAS, who have never learned R, and who produce brilliant work. The questions, the ideas, and the knowledge matter too, after all.
(*I should note that I'm a quantitative/theoretical ecologist, and I think every ecologist should have a strong quantitative education! Just that you can do that using lots of different methods and doing it well should always be the first priority.)
Thursday, June 11, 2015
The problem with collaboration in the electronic era...
E-communication has revolutionized every aspect of our lives. From how we shop, find love, watch movies and do science, the ability to interact with others globally has virtually eliminated barriers to the flow of ideas. I have fruitful collaborations with researchers in many different countries, which are greatly enhanced by e-mail and Skype. However, a new problem has emerged -scheduling people for meetings in multiple timezones!
I routinely have Skype meetings with my editorial team in the UK at 5 or 6 am, but as the above graphic shows -scheduling a meeting amongst people in the UK, North America and Australia is virtually impossible.
![]() |
Green = optional working time for researchers in different timezones; yellow = suboptimal; red = perhaps we allow people to sleep. |
Thursday, May 28, 2015
Are scientists boring writers?
I was talking with an undergrad who is doing her honours project with me about the papers she’s reading, and she mentioned how difficult (or at least slow going) she’s found some of them. The papers are mostly reviews or straightforward experimental studies, but I remember feeling the same way as an undergraduate. Academic science writing uses its own language, and until you are familiar with the terms and phrases and article structure, it can be hard going. Some areas, for example theoretical papers, even have their own particular dialects (you don’t see the phrase “mean-field approximation” in widespread usage, for example). Grad school has the advantage of providing total immersion into the language, but for many students, lots of time/guidance and patience is necessary to understand the primary literature. But is science necessarily a boring language?
A recent blog piece argues that academic science writing needs to fundamentally change because it is boring, repetitive, and uninspired. And as a result, the scientific paper needs to evolve. The post quotes a biologist at University of Amsterdam, Filipe Branco dos Santos: he feels that the problem is rooted in the conservative nature of scientists, leading them to replicate the same article structure over and over again. Journals act as the gatekeeper for article style too – submission requirements enforce the inclusion of particular sections (Introduction, Methods, Results, Discussion, etc), and determine every thing from word counts, figure number, text size, and even title structure and length. Reviewers and editors are within their rights to require stylistic changes. The piece includes a few tips for better article writing: choose interesting titles, write in the active tense, use short sentences, avoid jargon, include a lay summary. It’s difficult to disagree with those points, but unfortunately the article makes no attempt to suggest what, precisely, we should be doing differently. Still, it suggests that consideration of the past, present and future of scientific writing is necessary.
One glaring issue with the post is that the argument that scientists are stuck in a pattern established hundreds of years ago ignores just how much science papers have changed, stylistically. Scientific papers are a very old phenomenon – the oldest, Philosophical Transactions of the Royal Society, was first published in 1665. The early papers were not formatted in the introduction / methods / results / discussion style of today, and were often excerpts from letters or reports.
From the first issue, “Of the New American Whale-fishing about the Bermudas” begins:
“Here follows a relation, somewhat more divertising, than the precedent Accounts; which is about the new Whale-fishing in the West Indies about the Bermudas, as it was delivered by an understanding and hardy Sea-man, who affirmed he had been at the killing work himself.”
Ecological papers written in the early 1900s are also strikingly different in style than those today. Sentences are long and complex, words like “heretofore”, “therefore”, and “thus” find frequent usage, and the language is rather flowery and descriptive.
From a paper in the Botanical Gazette in 1913, the first sentence:
“Plant geographers and climatologists have long been convinced that temperature is one of the most important conditions governing the distribution of plants and animals, but very little has as yet been accomplished toward finding out what sort of quantitative relationships may exist between the nature of floral and faunal associations and the temperature conditions that are geographically concomitant therewith.”
While this opening makes perfect sense and establishes the question to be dealt with in the paper, it probably wouldn’t make it past review without comment.
Some of my favourite examples that highlight how much ecological papers have changed come from R.H. Whittaker’s papers. He is clearly an avid (and verbose) naturalist and his papers are peppered with evocative phrases. For example, “If, for example, one stands on a viewpoint in the Southern Appalachian Mountains in the autumn, one sees a complex varicoloured mantle of vegetation covering the mountain topography” and “The student of vegetation seeks to construct systems of abstraction by which relationships in this mantle of vegetation may be comprehended.” Indeed!
Today, in contrast, academic science writing is minimalist – it is direct, focused, and clarity is prized. Sentences are typically shorter, with a single focal thought, and the aim is for a clear narrative without the peripatetic asides common in older work. These shifts in style reflect the prevailing thoughts about how to balance the role of scientific papers as a communication device versus as a contribution to the scientific record. It seems that science papers may be boring now because authors and editors would rather a paper be a little dry rather than be unclear or difficult to replicate. (Of course, some papers manage to be both boring and confusing, so this is not always successful….) Modern papers have a lot of modern bells and whistles too. The move away from physical copies of papers to pdfs and online only colour versions and supplementary information has made sharing results easier and more comprehensive than ever.
If there is going to be a revolution in academic science writing, it will probably be tied to the ongoing technological changes in science and publishing. The technology is certainly already present to make science more interactive to the reader, which might make it less boring? It is already possible to include videos or gifs in online supplements (a great example being this puppet show explaining Diversitree). More seriously, supplements can include data, computer code used for analyses or simulations, additional results. It’s possible to integrate GitHub repositories with articles tied to a paper’s analyses, or link markdown scripts for producing manuscripts. The one limitation is that these approaches is that they aren’t included in the main text and so most people never see them. It’s only a matter of time before we move towards a paper format that includes embedded elements (extending on current online versions that include links to reference papers). One could imagine plots that could be manipulated, or interactive maps, allowing you to explore the study site through satellite images of the vegetation and terrain.
Increasingly interactive papers might make it more fun to work through a paper, but a paper must stand alone without them. For me, the key to a well-written paper is that there is always a narrative or purpose to the writing. Papers should establish a focus and ensure connections between thoughts and paragraphs are always obvious to the reader. The goal is to never lose the reader in the details, because the bigger picture narrative can be read between the lines. That said, I rarely remember if a paper is boringly written: I remember the quality of the ideas and the science. I would always take a paper with interesting ideas and average writing over a stylish paper with no substance. So perhaps academic science writing is an acquired (or learned) taste, and certainly that taste could be improved, but it's clear that science writing is constantly evolving and will continue to do so.
Wednesday, May 20, 2015
I'll take 'things that have nothing to do with my research' for $400
I guess I do have a couple papers with the word fire in their titles?
And to Burns and Trauma's credit, this is a nicely formatted email and the reasons to publish with them are pretty convincing :-)
Monday, April 20, 2015
The wonder(ful) years? Being a postdoc.
A surprising number of academics talk about their postdocs the same way some people talk about high school – as the best time of their life. It’s enough to make you feel like you’re doing something wrong if you don’t feel the same way :). In fact, postdoc jobs vary greatly and are usually a bit more complex than the “wonderful time to do the research you love and have few responsibilities” memories.
The truth is, every postdoc position and postdoctoral fellow is different, and each has its own strengths and weaknesses. Not every postdoc will be a glorious NCEAS position--self-directed, collaborative, community-oriented (and in Santa Barbara). And not every postdoc will be part of a funded, predetermined research project where you are just a cog in the lab-machine. (Though some people would legitimately prefer the latter to the former).
There are many great aspects to being a postdoc. After a PhD, you no longer need to take classes, you may not need to teach, and research is finally your primary focus. Even better, ‘the thesis’ isn’t looming over your head. And usually you make actual money, rather than poverty-level grad student wages, even if the actual amount is modest. Finally, you probably don’t have nearly as many responsibilities as the average tenured or tenure-track academic (and so, the assumption is, your stress levels are better). (And for those who need a little ego boost, you occupy a slightly better position in the lab hierarchy, maybe you get a better office and get a little more respect.) Best of all, you can finally stop saying you’re a student.
These are all wonderful in theory, but sometimes the reality is more complex. Because academic positions, including postdocs, are hard to find, not everyone will be able to land a position that is well-matched to their skills and research interests. This can feel frustrating, since academics in general want intellectual stimulation and skill development. Finding the perfect lab is difficult, and finding the perfect lab with money to pay you is even harder. As a result, that perfect path on the CV from PhD to postdoc lab where you expand your skills or fine-tune your interests is exceptional.
For many people, the postdoc is a time with a large set of associated stresses; first and foremost, “what’s next?” (for you, your family, your career, your geographic position on the earth...). This is the period when the next position and, more generally, your career, is at the forefront. And the timeframe in which you must sort everything out is short, since most postdoc positions last only 1-3 years. It is not uncommon to run out of funding before the next position has been acquired. Get a group of postdocs together in a room, and the undercurrent of worry will be palpable.
And of course, the short length of most postdoc jobs means that you will probably have to move more frequently than ever. Combined with the unorganized nature of postdoc labour, this can make for a lonely time. In smaller departments, postdocs are few and transient, making it difficult to feel part of a community. No longer a student, not quite a faculty, only temporarily in a place, it can be hard to find a sense of belonging.
None of this is to bemoan the postdoc life, just to note that as with all things, it has pros and cons. I like being a postdoc. I’ve also been lucky to have independent funding though, which no doubt has made things easier. Still, the upsides have included the ability to developing a research plan for the long term (and to make mistakes and fail while the stakes are still low), to supervise undergraduates, to develop a new skills or viewpoints, and definitely to have time for manuscript writing. But I do hope that these aren’t the *best* research years of my life, because—like high school—things can always improve.
The truth is, every postdoc position and postdoctoral fellow is different, and each has its own strengths and weaknesses. Not every postdoc will be a glorious NCEAS position--self-directed, collaborative, community-oriented (and in Santa Barbara). And not every postdoc will be part of a funded, predetermined research project where you are just a cog in the lab-machine. (Though some people would legitimately prefer the latter to the former).
There are many great aspects to being a postdoc. After a PhD, you no longer need to take classes, you may not need to teach, and research is finally your primary focus. Even better, ‘the thesis’ isn’t looming over your head. And usually you make actual money, rather than poverty-level grad student wages, even if the actual amount is modest. Finally, you probably don’t have nearly as many responsibilities as the average tenured or tenure-track academic (and so, the assumption is, your stress levels are better). (And for those who need a little ego boost, you occupy a slightly better position in the lab hierarchy, maybe you get a better office and get a little more respect.) Best of all, you can finally stop saying you’re a student.
These are all wonderful in theory, but sometimes the reality is more complex. Because academic positions, including postdocs, are hard to find, not everyone will be able to land a position that is well-matched to their skills and research interests. This can feel frustrating, since academics in general want intellectual stimulation and skill development. Finding the perfect lab is difficult, and finding the perfect lab with money to pay you is even harder. As a result, that perfect path on the CV from PhD to postdoc lab where you expand your skills or fine-tune your interests is exceptional.
For many people, the postdoc is a time with a large set of associated stresses; first and foremost, “what’s next?” (for you, your family, your career, your geographic position on the earth...). This is the period when the next position and, more generally, your career, is at the forefront. And the timeframe in which you must sort everything out is short, since most postdoc positions last only 1-3 years. It is not uncommon to run out of funding before the next position has been acquired. Get a group of postdocs together in a room, and the undercurrent of worry will be palpable.
And of course, the short length of most postdoc jobs means that you will probably have to move more frequently than ever. Combined with the unorganized nature of postdoc labour, this can make for a lonely time. In smaller departments, postdocs are few and transient, making it difficult to feel part of a community. No longer a student, not quite a faculty, only temporarily in a place, it can be hard to find a sense of belonging.
None of this is to bemoan the postdoc life, just to note that as with all things, it has pros and cons. I like being a postdoc. I’ve also been lucky to have independent funding though, which no doubt has made things easier. Still, the upsides have included the ability to developing a research plan for the long term (and to make mistakes and fail while the stakes are still low), to supervise undergraduates, to develop a new skills or viewpoints, and definitely to have time for manuscript writing. But I do hope that these aren’t the *best* research years of my life, because—like high school—things can always improve.
Tuesday, March 10, 2015
Scientific Presentations: the Dos and Don'ts
www.pixshark.com
With the ESA submission deadline just passing, the Cadotte
Lab decided that it would be helpful to dish out a few tips on how to make a
presentation that is both enjoyable for your audience and fun for you to give. Presenting
in front of people is never easy; giving a presentation about your own study
can be even harder since you have to condense months (or even years) worth of
information into a 15 minute time period. So with this in mind here are a few
tips for each of the main sections of a presentation:
Note, the percentage by each section heading indicates the
relative amount of time you should spend on that section.
Title Slide (5%)
www.nichecartoons.com
This is the first chance you’ll get to catch your audience’s
attention, so be interesting!
The title of your presentation depends on the type of
audience you’ll be presenting to, so gauge it accordingly. If your audience is
a bunch of people with only general biology backgrounds or people that are from
completely different fields then don’t complicate things using heavy jargon.
Generally for the title, you want to:
- Be witty and interesting
- Convey the main message or main result from your study
If you’re speaking to a broad audience it could be helpful
to have a broad title and then separate it from a more specific title.
Besides the title you’ll also want to include your name and
affiliation. Depending on the type of talk, for instance an honors thesis, you
should also include your supervisor’s name. If you are collaborating with many
people on a study you should also include their names. However, make sure that
your name is on the first slide, since you are the presenter, and then on a
second slide include a special acknowledgement of the other people involved.
It’s also recommended that you acknowledge these people throughout the talk,
such as in the methods.
Introduction (10-15%)
Don’t make this section too long. Give just enough
background that the audience can understand the concepts that you’ll be
discussing and how it relates to the question you are trying to answer.
Generally for the introduction, you want to:
- Have the background information displayed in a simple to understand way
- You could use info-graphs here to reinforce an idea
- By the 2nd or 3rd slide you’ll want to state your study objectives or hypotheses
- You could create ‘toy’ graphs to describe your hypotheses / predictions
Methods (10-15%)
Be very concise with this section. Everyone understands that
a lot of work went into performing your study; however, you don’t want to
overwhelm your audience with all the nitty-gritty things you had to do. Give
enough detail that people understand what you did and if possible try and
summarize your methods in a simple figure.
Generally for the methods, you want to talk about:
- The treatments used, sample size, the measurements taken and how they were done, and the statistics that you performed
A note on statistics: try to steer clear of very complicated
statistics. Most likely your audience will have a basic understanding of stats,
but you may lose people if you get too complicated. When talking about your
stats, make sure that you can give an easy to understand explanation of how
they work.
Results (50%)
This is the biggest and best section; it’s where you get to
show people all the cool and exciting things you’ve done! However, the only way
you can convey how awesome your results are is by clearly explaining them.
Generally for the results, you want to:
- Stick to the main results
- You may have a lot different results but always make sure that what you are describing relates directly to the main message of your study
- Don’t overwhelm your audience
- Always thoroughly describe your graphs
- Describe what variables were you examining (the axes)
- Why is the graph important?
- What is the relationship that the graph is showing?
- The title of the slide could be used to state what the result is
- You’ve spent a lot of time making these graphs and analyzing them - so you know them very well, but your audience doesn’t yet. Take time to walk them through the graphs.
- If you’re showing several graphs in sequence, make sure to note if the axes are changing
- If the graphs are very similar it might be helpful to have a break between slides or to use an animation.
- Don’t show too many stats
- Just state the p-values and which stats were used
- Avoid tables if possible
- Summarize all the information in an easy to follow figure
- If you can’t avoid using a table make it as appealing as possible
- Highlight key parts or add arrows to show trends if they exist
Discussion (20%)
Now start bringing everything back together. Your audience
may have gotten lost during your results section, so now is the time to refocus
them so that they can see the big picture.
Generally for the discussion, you want to:
- Restate your hypotheses
- Restate you main results
- Describe how you could improve your study
- Describe the next steps for your work and the field in general
In the end you’ll want to describe the broader implications
of your work and give the audience a take home message so that they know that
your work is bettering the field in some way.
Acknowledgements (5%)
Don’t forget to thank everyone who has helped you through
this whole process! This includes your supervisor, people who helped you with
data analysis or revising your paper, or all the volunteers you helped you
conduct your field work or lab work. You’ll also want to acknowledge your
institution as well as anyone who provided funding to your project.
General tips
Here’s a quick list of tips to use throughout your presentation:
- Use large text font
- Don’t be flashy, make sure it’s easy to read
- Don’t put too much text on a slide
- This distracts the audience
- Don’t put any important point (text or an image) at the bottom 1/3rd of a slide
- Depending on the room you are presenting in it may be very hard for the audience to see it
- In general, try and keep everything within the top 2/3rd of the slide
- Don’t put too many animations on a slide
- This can be very distracting for the audience
- Don’t read off your slides
- Use presenter view if you can’t memorize everything
- Including outlines
- Not necessary in a short talk, but could be helpful in a longer talk
- If you run out of time
- Panic on the inside not the outside!
- Acknowledge that you’re running out of time and start wrapping things up
- Start talking about the broad implications of your work and maybe future directions you plan to take
- If you have more slides, skip over them but tell the audience what you were planning on showing. If they ask questions about what you were going to show you can go back to those slides
- Don’t talk too fast!
- Everyone gets nervous! Take a deep breath and calm yourself down, the calmer you are the easier it is for your audience to follow you
Monday, March 9, 2015
In praise of difficult questions.
There were a lot of people at my graduate institution who weren’t afraid to ask probing, thoughtful, difficult questions. They asked them seemingly without any concern about making the recipient feel bad, although students were more likely to receive kinder versions, and they asked them at departmental talks, committee meetings, student seminars, and at faculty interviews. I’ll admit there were times when this made me uncomfortable, and it certainly contributed no small amount of anxiety before giving talks there (and I’m sure I’m not the only person who felt that way).
These days I find myself missing those tough questions, not because I enjoy confrontation per se, but because they made an important contribution to my education.
These days I find myself missing those tough questions, not because I enjoy confrontation per se, but because they made an important contribution to my education.
To be clear, bullying questions or competitive questioning meant to highlight the questioner’s intelligence are a waste of time (e.g. two minutes of talking about your research followed by "what do you think about that?"). Critical thinking, while one of the most important aspects of a post-graduate education, can't be taught. But tough questions and questioners model critical thinking for students in the most direct way. Being at the front of the room talking does not automatically grant expert status: the speaker's ideas must be clear and robust to debate.
Difficult questions benefit a speaker too - they are the clearest demonstration that the audience has engaged with their work. The most useful talks are those in which the questions are thought provoking for both the speaker and the audience.
And finally, it can be refreshing when a questioner holds a person to actually answering the question. Science is built on debate and some times disagreement. Hard questions made me feel that the people asking them were expressing a preference for good science, even if the cost was some discomfort or social unease. And that feels like an important thing to express.
Friday, March 6, 2015
Distilling an ocean of theory and adding a few of your own drops
I recently
completed my PhD qualifying exam at the University of Toronto-Scarborough for
the Department of Physical and Environmental Science. Prior to going through the
process the exam took on a sort of “black box” quality where I’d seen colleagues
pass through unscathed but the depth of questioning that took place during the
oral examination remained unclear. So I thought it might be of some value to
comment on my experience with the process.
The format of these exams is fairly variable across departments and between institutions with some requiring the production of several essays in a short period of time, some based on an extensive readings list, some formatted as a proposal defense and others including some or all of these components. My exam took the form of a proposal defense which required submitting a 9000-word proposal outlining the theoretical framework & justifications for my research questions, hypotheses, objectives, methodologies, preliminary results, discussion and thoughts on the significance of the work, a 25-minute presentation of this proposal followed by an oral examination that lasted about an hour and 30 minutes. These exams are typically meant to be taken at the early stages of one’s PhD, but it seems that they often get kicked further down the road, as was the case with mine which I completed half way into my 3rd year of a 5 year program. This had its advantages and disadvantages where further progress allowed presentation and discussion of some interesting findings and a clearer picture of what my thesis is going to look like, but also came with the colossal challenge of organizing everything into what seemed like a miniscule 25-minute presentation. This was probably the most challenging academic exercise I have faced.
I finalized my presentation a few days before my exam, and felt that it had a nice balance between theory and my contributions, but this only after “throwing away” 100+ slides in the 2 weeks leading up to the exam… And while that might sound like a total waste of time, it actually forced me to distill what seemed like an “ocean of theory” to the essential elements that grounded my work. Further, developing slides that can visually communicate complex theory is a great form of study that can serve you well during the oral exam; even if you can’t show the slides you will know the material. Also, I can’t overstate the importance of peer and supervisory assistance here. I was extremely lucky to have my presentation lovingly torn to shreds by my lab mates. This can be a terrifying process as we know that imposter syndrome is alive and well in academia (http://irblog.eu/impostor-syndrome-phd/). Yet, we of course survive these practice talks and our presentations benefit greatly.
Once I was happy with the content and flow of my talk I decided to inject a little humour by photoshopping some images and spattering in a couple silly animations. This was probably some kind of self-defense mechanism where I was hoping that by putting a smile on the face of an examiner I might be able to ease my own nerves and the general tension that goes along with a comprehensive exam. Of course, whether this succeeds or not will depend on the demeanor of your examiners, your delivery and probably the general quality of the rest of the presentation. In my case, I found that the humour worked and offered a nice lull in the tension. I highly recommend trying this, once you’ve nailed down the meat of the talk of course. Beyond attempts at humour, you should know the talk. You shouldn’t be reading off any notes and should only read out points on the slide that are essential theory items or specific research questions, hypotheses or findings. There will be an upcoming blog post on presentation tips, so I’ll stop there… Just remember that in this exam, your presentation sets the tone. It is your opportunity to articulate your comprehension of the subject and the novelty of your work.
The format of these exams is fairly variable across departments and between institutions with some requiring the production of several essays in a short period of time, some based on an extensive readings list, some formatted as a proposal defense and others including some or all of these components. My exam took the form of a proposal defense which required submitting a 9000-word proposal outlining the theoretical framework & justifications for my research questions, hypotheses, objectives, methodologies, preliminary results, discussion and thoughts on the significance of the work, a 25-minute presentation of this proposal followed by an oral examination that lasted about an hour and 30 minutes. These exams are typically meant to be taken at the early stages of one’s PhD, but it seems that they often get kicked further down the road, as was the case with mine which I completed half way into my 3rd year of a 5 year program. This had its advantages and disadvantages where further progress allowed presentation and discussion of some interesting findings and a clearer picture of what my thesis is going to look like, but also came with the colossal challenge of organizing everything into what seemed like a miniscule 25-minute presentation. This was probably the most challenging academic exercise I have faced.
I finalized my presentation a few days before my exam, and felt that it had a nice balance between theory and my contributions, but this only after “throwing away” 100+ slides in the 2 weeks leading up to the exam… And while that might sound like a total waste of time, it actually forced me to distill what seemed like an “ocean of theory” to the essential elements that grounded my work. Further, developing slides that can visually communicate complex theory is a great form of study that can serve you well during the oral exam; even if you can’t show the slides you will know the material. Also, I can’t overstate the importance of peer and supervisory assistance here. I was extremely lucky to have my presentation lovingly torn to shreds by my lab mates. This can be a terrifying process as we know that imposter syndrome is alive and well in academia (http://irblog.eu/impostor-syndrome-phd/). Yet, we of course survive these practice talks and our presentations benefit greatly.
Once I was happy with the content and flow of my talk I decided to inject a little humour by photoshopping some images and spattering in a couple silly animations. This was probably some kind of self-defense mechanism where I was hoping that by putting a smile on the face of an examiner I might be able to ease my own nerves and the general tension that goes along with a comprehensive exam. Of course, whether this succeeds or not will depend on the demeanor of your examiners, your delivery and probably the general quality of the rest of the presentation. In my case, I found that the humour worked and offered a nice lull in the tension. I highly recommend trying this, once you’ve nailed down the meat of the talk of course. Beyond attempts at humour, you should know the talk. You shouldn’t be reading off any notes and should only read out points on the slide that are essential theory items or specific research questions, hypotheses or findings. There will be an upcoming blog post on presentation tips, so I’ll stop there… Just remember that in this exam, your presentation sets the tone. It is your opportunity to articulate your comprehension of the subject and the novelty of your work.
The written component of the proposal, on the other hand, can seem to be propelled by a perpetual motion machine generating an endless sprawl of “conceptual axes”, “synthetic approaches” and “novel perspectives” about your thesis topic. Here, you can definitely produce a fairly comprehensive picture of the subject and your perspectives but you’ll still have to tug the reigns so as not to irritate your readers with a bloated document. If you find yourself delving into the linkages between your thesis and systems theory, and you’re not in physics, odds are you’ve gone too far. Everything in your written proposal is essentially fair-game for the oral examination, so don’t let it disappear from your desktop once you’ve submitted it. You will most certainly get questions about the methods you’ve proposed or have employed, and you will need to be able to justify your choices and situate your studies within the literature.
The oral examination will surely be one of the most unnerving experiences of your academic life, but you can minimize your unease by continually drawing those links between your thesis and the literature in the weeks leading up to the exam. I found the oral exam to be a very fair process where I was tested on the biophysical interactions that I was examining, the measures that I used, and the conceptual links between my thesis components and the trends in the literature. Now, my thesis is fairly atypical in that it takes a multi-disciplinary approach to a larger topic, and this definitely generated some questions about the linkages between the various components. But beyond that challenge I think any questions about the “grand scheme” of your thesis can be addressed by highlighting those initial motivations that you included in your application to your program. In my application, I was required to write a page about why interdisciplinary perspectives are essential in the field of environmental science, and I was able to pull from that motivation to answer these kinds of questions. Odds are that your initial reasons for engaging with a certain research topic will ground a lot of your answers during the oral examination. One question that I didn’t anticipate was essentially “where do you see yourself in 10 years”? I think in our PhD’s we can easily get tunnel vision and forget that there is an end to the process at which point we’ll move on to something new. So don’t forget about that light at the end of the tunnel during the exam. Think about your future aspirations and how far you’ve come since you became fascinated with your topic. Your examiners want to feel that engagement and passion. And you will get questions about the theory that are right in your wheelhouse, so take advantage when they appear and highlight both your understanding of the unanswered questions and how your work is not just adding to the complexity but is helping to bridge those gaps.
In the end, after all the late nights of writing, pecking at bowls of nuts (because cooking takes too long) and re-arranging your presentation slides for the 100th time, you’ll most likely find that this process has probably been the most constructive thing that you’ve ever been a part of.
Wednesday, March 4, 2015
Graduate students- employees, scholars, or something in between?
Graduate school has always required that students balance research, classwork, and teaching activities (perhaps with some time for complaining). Though many aspects of graduate school are unchanged, there can be a tension between grad students and their employers driven by a shift in both these groups’ expectations, and the complex nature of STEM graduate school.
The real truth about graduate school according to the Simpsons...
This is illustrated well by the current strikes of teaching assistants (primarily graduate students) at University of
Toronto and York University – both major Canadian institutions. [And even more
extreme cases exist]. The union at U of T has become a defacto
union for graduate student issues as well, and the primary sticking point
appears to be graduate student stipends, which are far below the poverty line. The
students there are striking as teaching assistants (so research work can
continue) but their main issue is a holistic “graduate student”
issue.
Supposing the components of graduate school have remained similar over the years, why might tension be increasing between what graduate students and faculty/departments expect? Partly because so many other things have changed-–the economy, the
workforce, cultural expectations. I think that in the past, it was easier to
consider graduate school as a place of passion and intellectual curiosity, where
one would make a lousy salary, but consider it “worth it”. Today, the cost-benefit analysis for getting a PhD is
considerably less positive – it takes longer to get a PhD, on average, and the payoff in terms of obtaining a faculty or other job, makes this less clear. The cost of education, particularly in the US, is immense: the possibility of student loan debt from 4-8 years of postgraduate education is fairly unpalatable.
From Nature. |
As the realities change, so too do the expectations. That on its own would be the source of some tension. But the dual nature of graduate school compounds the tensions since it is difficult for graduate students, faculty, and department heads to evaluate what reasonable expectations are for things such as pay, hours, vacation time. For most students, graduate school has aspects of both a clear job (usually teaching duties—running labs, marking tests and assignments, sometimes lecture duties) and a clear studentship (class work, appraisal exams, all culminating in a defense). It also includes research, done in a lab or the field, which may vary between being a job (doing tasks primarily for the PI, monitoring undergrads, ordering supplies) and an intense learning experience. Employment involves contracts with expectations and restrictions, set hours and wages; being a student lacks the same expectations but is often associated with greater freedom and personal growth. The extent to which faculty and graduate students see the position as “student” or as “job” may well differ.
The interaction of economic realities with the duality of graduate school is an important issue. Should graduate school be considered the start of one's working life? If so, is it equivalent to an entry-level position? After all, TAs do a lot of grunt work -- marking, marking, and more marking, run simple labs and tutoring sessions -- and many universities hire undergraduates to do similar tasks. On the other hand, graduate students are also high-achievers doing complicated analyses for research, and have reasonably high education levels. Graduate school may come with opportunity costs - peers with similar educations tend to have jobs and retirement funds. In contrast, the pure academic path usually means you will live frugally for many years before your first "real" position (and you may be in your 30s or later before you get it).
There may be some generational changes as well. It is suggested that Millenials/Generation Y have different priorities than previous generations: they strongly desire fulfilment from their work, but also competitive compensation and job flexibility (e.g.). The downsides of graduate school are greater and perhaps more obvious to this generation: if it is a job, it is poorly paid and entry-level, if it is a studentship, it comes with an opportunity cost. But how to evaluate it when it is both? It is undeniably easier to go through graduate school for those who don't have to deal with the dualities - such as through having a fellowship that allows a student to do research and classes only. Most people are still in graduate school for the same reasons as they always have been - love of science and learning. That hasn't changed. But the meaning of graduate school itself may well have changed. There is no one or easy solution to the issue. But no doubt a recognition by both sides of the realities of being a graduate student (and a supervisor) and honest communication about expectations on both sides (and sometimes, perhaps a little pressure) would go far.
The interaction of economic realities with the duality of graduate school is an important issue. Should graduate school be considered the start of one's working life? If so, is it equivalent to an entry-level position? After all, TAs do a lot of grunt work -- marking, marking, and more marking, run simple labs and tutoring sessions -- and many universities hire undergraduates to do similar tasks. On the other hand, graduate students are also high-achievers doing complicated analyses for research, and have reasonably high education levels. Graduate school may come with opportunity costs - peers with similar educations tend to have jobs and retirement funds. In contrast, the pure academic path usually means you will live frugally for many years before your first "real" position (and you may be in your 30s or later before you get it).
There may be some generational changes as well. It is suggested that Millenials/Generation Y have different priorities than previous generations: they strongly desire fulfilment from their work, but also competitive compensation and job flexibility (e.g.). The downsides of graduate school are greater and perhaps more obvious to this generation: if it is a job, it is poorly paid and entry-level, if it is a studentship, it comes with an opportunity cost. But how to evaluate it when it is both? It is undeniably easier to go through graduate school for those who don't have to deal with the dualities - such as through having a fellowship that allows a student to do research and classes only. Most people are still in graduate school for the same reasons as they always have been - love of science and learning. That hasn't changed. But the meaning of graduate school itself may well have changed. There is no one or easy solution to the issue. But no doubt a recognition by both sides of the realities of being a graduate student (and a supervisor) and honest communication about expectations on both sides (and sometimes, perhaps a little pressure) would go far.
The real truth about graduate school according to the Simpsons...
**I just want to note that this is inspired by--but not addressing--the U of Toronto situation, and any comments that simply want to debate specific circumstances in particular universities will be deleted...
Larger discussion of the general issue always welcome.
Thursday, February 12, 2015
Darwin in images (Darwin Day 2015).
A famous Vanity Fair caricature from 1971.
The oft-repeated mantra that evolution means that man evolved directly from a monkey or beast was an early (and still popular) theme. Darwin often took the role of the monkey.
![]() |
John Tenniel for Fun magazine (1872) |
![]() |
From 1882, Punch’s Almanack, Linley Sambourne |
(Link)
Even in his day, some cartoons supported rather than poked fun. See the speech on the wall, a plea avoid ignorance of Science. (Link)
And finally, Darwin's own images have been incredibly influential. He was a talented naturalist and scientist and left many lovely illustrations. His sketches in his books, particularly the "tree of life" image has become an emblem for many scientists - of evolution and the origin of species, of immense intellectual accomplishment, of the birth of modern ecology and evolution.
To learn more about Darwin in images, this is a great resource.
Even in his day, some cartoons supported rather than poked fun. See the speech on the wall, a plea avoid ignorance of Science. (Link)
![]() |
"Puck Presents Archdeacon Farrar’s New Year’s Hint — A Needed Course of Instruction for Our Religious Instructors"(1890). |
The "evolution of man" meme has a long history - what was originally satire is primarily now a visual joke. (Link)
![]() |
Harper's Bazaar 1871 |
![]() |
Modern concerns. |
(Link)
Darwin and evolution has been a repeated image in US politics, covering evolution and education, religious tension, science, and social darwinis, among other themes. (Link)
![]() |
1925 SF Examiner |
![]() |
More recent, by Karl Wimer |
Religion and Darwin have been unavoidable companions.
From pro-religion angles:
![]() |
1922 Moody's bible institute |
And anti-religious:
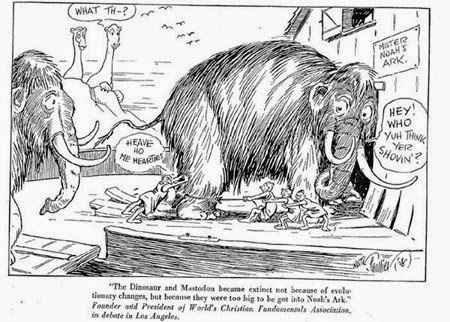
Darwin pops up on motivational images and posters:
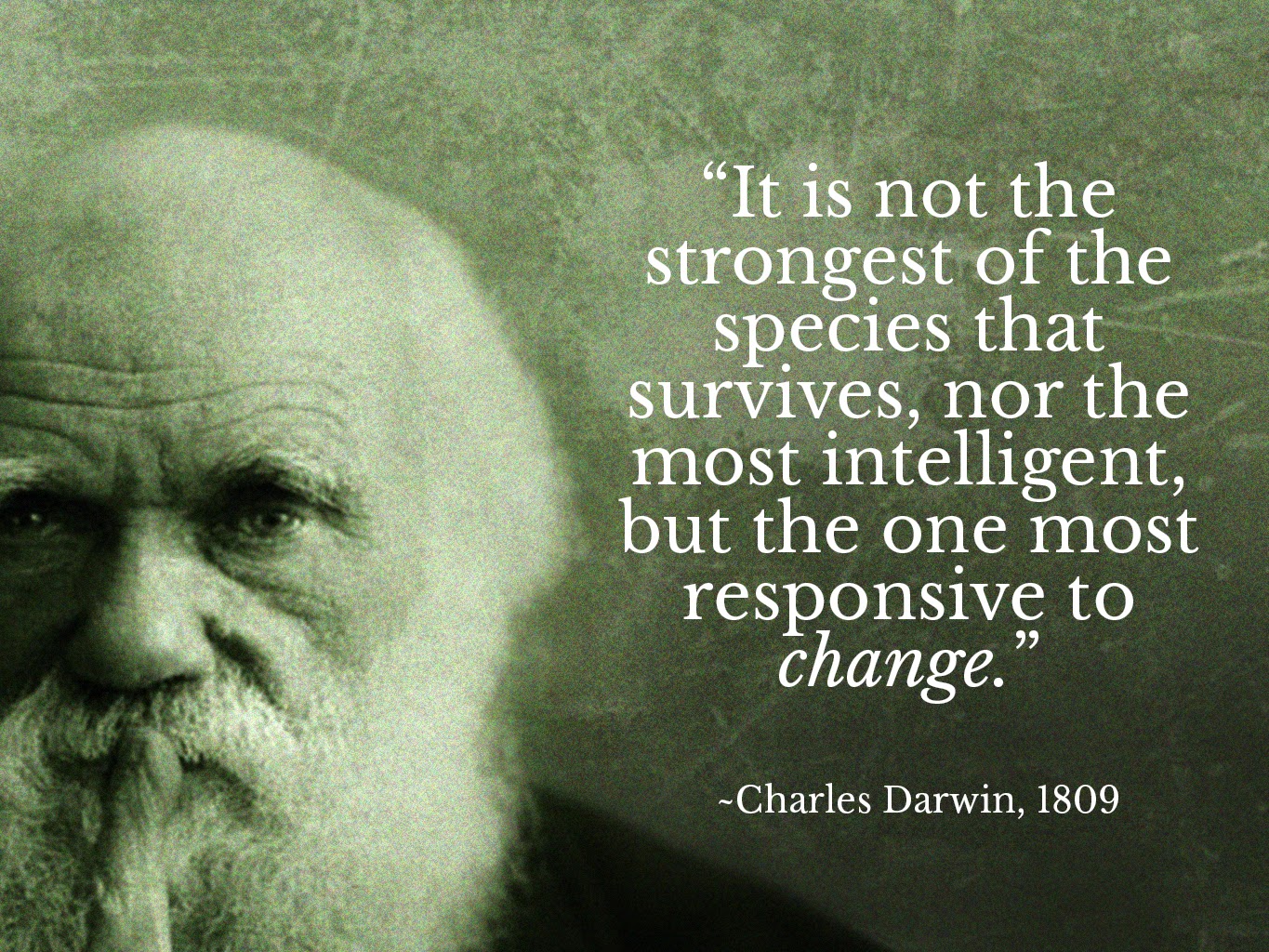
And evolution jokes remain eternally popular:
![]() |
The Farside providing one of the better ones :) |
![]() |
source unknown |
![]() |
1983 |
![]() |
The famous "I think" image - the tree of life. |
To learn more about Darwin in images, this is a great resource.
Tuesday, February 3, 2015
Predatory open access journals: still keep'n it classy
As most academics are aware, there are hundreds of predatory open access journals that try to trick authors into submitting to their journals, charge exorbitant fees, and do not ensure that articles are peer reviewed or live up to basic scientific standards. The most celebrated cases are journals that embarrassingly publish non-sensical fake papers. I don't know why, but I sometimes go to the journal websites to see what they publish or who is on their editorial boards. I received such an e-mail this morning from SOJ Genetic Science published by Symbiosis, a recognized predatory publisher. This journal, unlike others, actually has a single published issue with an editorial! I thought: "wow, are they trying to be legitimate?"; then I read the editorial. The editorial is probably best described as a nonsensical diatribe about genetics, which lacks any real connection to modern genetic theory. Here is my favourite paragraph:
Predatory open access journals: still keep'n it classy.
Predatory open access journals: still keep'n it classy.
Monday, October 6, 2014
What is ecology’s billion dollar brain?
(*The topic of the billion dollar proposal came up with Florian Hartig (@florianhartig), with whom I had an interesting conversation on the idea*)
The first thing I recognized was that in proposing the 'ultimate' ecological project, you're implicitly stating how you think ecology should be done. For example, do you could focus on the most general questions and start from the bottom. If this is the case, it might be most effective to ask a single fundamental question. It would not be unreasonable to propose to measure metabolic rates under standardized conditions for every extent species, and develop a database of parameter values for them. This would be the most complete ecological database ever, that certainly seems like an achievement.
But perhaps you choose something that is still of general importance but less simplistic, and run a standardized experiment in multiple systems. This has been effective for the NutNet project. Propose to run replicate experiments with top-of-the-line warming arrays on plant communities in every major ecosystem. Done for 10 years, over a reasonably large scale, with data recorded on physiology and important life history events, this might provide some ability to predict how warming temperatures are affecting ecosystems.
The alternative is embrace ecological complexity (and the ability to deal with complexity that 1 billion dollars offers). Given the analytic power, equipment, and man hours that 1 billion dollars can buy, you could record every single variable--biotic, abiotic, weather--in a particular system (say, a wetland) for every second of every day. If you don’t simply drown in the data you’ve gathered, maybe you can reconstruct that wetland, predict every property from the details. While that may seem a bit extreme, if you are a complexity-fatalist, you start to recognize that even the general experiments are quickly muddied by complexity. Even that simple, general list of species' metabolic parameters quickly spirals into complexity. Does it make sense to use only one set of standardized conditions? After all, conditions that are reasonable for a rainforest tree are meaningless for an ocean shark or a tundra shrub. Do you use the mean condition for each ecosystem as the standard, knowing that species may only interact with the variance or extremes in those conditions (such as desert annuals that bloom after rains, or bacteria that use cyst stages to avoid harsh environments). What about ontogenetic or plastic differences? Intraspecific differences?
It's probably best then to realize that there is no perfect ecological experiment. The interesting thing about the Human Brain project is that neuroscience is more like ecology than many scientific fields - it deals with complex organic systems with emergent properties and great variability. What ecology needs, ever so simplistically, is more data and better models. Maybe, like neuroscience, we should request a supercomputer that could located and incorporate all ecological data ever collected, across fields (natural history, forestry, agronomy, etc) and recognize the connections between that data, based on geography, species, or scale. This could both give us the most sophisticated possible data map, showing where the data gaps exist, and where areas are data-rich and ready for model development. Further, it could (like the Human Brain) begin to develop models for the interconnections between data.
It's probably best then to realize that there is no perfect ecological experiment. The interesting thing about the Human Brain project is that neuroscience is more like ecology than many scientific fields - it deals with complex organic systems with emergent properties and great variability. What ecology needs, ever so simplistically, is more data and better models. Maybe, like neuroscience, we should request a supercomputer that could located and incorporate all ecological data ever collected, across fields (natural history, forestry, agronomy, etc) and recognize the connections between that data, based on geography, species, or scale. This could both give us the most sophisticated possible data map, showing where the data gaps exist, and where areas are data-rich and ready for model development. Further, it could (like the Human Brain) begin to develop models for the interconnections between data.
Without too many billion dollar calls going on, this is only a thought experiment, but I have yet to find someone who had an easy answer for what they would propose to do (ecologically) with 1 billion dollars. Why is it so difficult?
Monday, September 15, 2014
Links: Reanalyzing R-squares, NSF pre-proposals, and the difficulties of academia for parents
First, Will Pearse has done a great job of looking at the data behind the recent paper looking at declining R and p-values in ecology, and his reanalysis suggests that there is a much weaker relationship between r2 values and time (only 4% rather than 62% as reported). Because the variance is both very large within-years and also not equal through time, a linear model may not be ideal for capturing this relationship.
Thanks @prairiestopatchreefs for linking this.
From the Sociobiology blog, something that most US ecologists would probably agree on: the NSF pre-proposal program has been around long enough (~3 years) to judge on its merits, and it has not been an improvement. In short, pre-proposals are supposed to use a 5 page proposal to allow NSF to identify the best ideas and then invite those researchers to submit a full proposal similar to the traditional application. Joan Strassman argues that not only is this program more work for applicants (you must write two very different proposals in short order if you are lucky to advance), it offers very few benefits for them.
The reasons for the gender gap in STEM academic careers gets a lot of attention, and rightly so given the continuing underrepresentation of women. The demands of parenthood often receive some of the blame. The Washington Post is reporting on a study that considers parenthood from the perspective of male academics. The study took an interview-based, sociological approach, and found that the "majority of tenured full professors [interviewed] ... have either a full-time spouse at home who handles all caregiving and home duties, or a spouse with a part-time or secondary career who takes primary responsibility for the home." But the majority of these men also said they wanted to be more involved at home. As one author said, “Academic science doesn’t just have a gender problem, but a family problem...men or women, if they want to have families, are likely to face significant challenges.”
On a lighter note, if you've ever joked about PNAS' name, a "satirical journal" has taken that joke and run with it. PNIS (Proceedings of the Natural Institute of Science) looks like the work of bored post-docs, which isn't necessarily a bad thing. The journal has immediately split into two subjournals: PNIS-HARD (Honest and Real Data) and PNIS-SOFD (Satirical or Fake Data), which have rather interesting readership projections:
Thanks @prairiestopatchreefs for linking this.
From the Sociobiology blog, something that most US ecologists would probably agree on: the NSF pre-proposal program has been around long enough (~3 years) to judge on its merits, and it has not been an improvement. In short, pre-proposals are supposed to use a 5 page proposal to allow NSF to identify the best ideas and then invite those researchers to submit a full proposal similar to the traditional application. Joan Strassman argues that not only is this program more work for applicants (you must write two very different proposals in short order if you are lucky to advance), it offers very few benefits for them.
The reasons for the gender gap in STEM academic careers gets a lot of attention, and rightly so given the continuing underrepresentation of women. The demands of parenthood often receive some of the blame. The Washington Post is reporting on a study that considers parenthood from the perspective of male academics. The study took an interview-based, sociological approach, and found that the "majority of tenured full professors [interviewed] ... have either a full-time spouse at home who handles all caregiving and home duties, or a spouse with a part-time or secondary career who takes primary responsibility for the home." But the majority of these men also said they wanted to be more involved at home. As one author said, “Academic science doesn’t just have a gender problem, but a family problem...men or women, if they want to have families, are likely to face significant challenges.”
On a lighter note, if you've ever joked about PNAS' name, a "satirical journal" has taken that joke and run with it. PNIS (Proceedings of the Natural Institute of Science) looks like the work of bored post-docs, which isn't necessarily a bad thing. The journal has immediately split into two subjournals: PNIS-HARD (Honest and Real Data) and PNIS-SOFD (Satirical or Fake Data), which have rather interesting readership projections:
Subscribe to:
Posts (Atom)